In this constantly changing sphere of software development, moving forward means innovating. This is where AI and Machine Learning come into the picture to revamp the entire game of QA metrics and software testing.
These technologies promise to bring change to the habit with which testing is practiced, leading to more efficient, accurate, and reliable processes.
So, what does it really mean for AI and Machine Learning in QA metrics? Let's dig deeper into their profound effects on our industry.
Introduction to AI and Machine Learning in the Software Testing Landscape
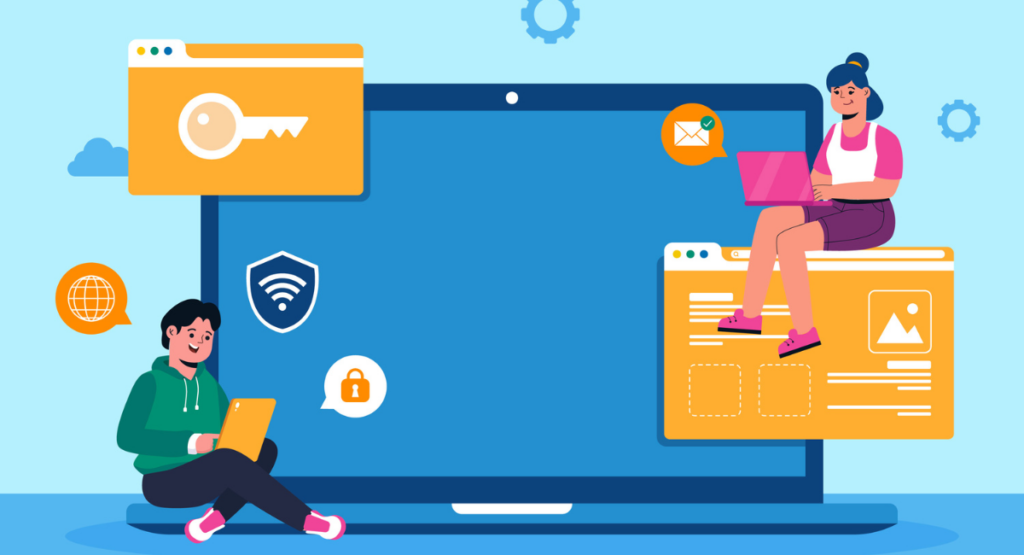
AI and Machine Learning are no more buzz and are integral to the software testing landscape. Thanks to these two, automation of repetitive tasks and predictive analytics, the testing processes are really effective.
This ensures less manual work for QA engineers and developers, with only the project manager left to spend time on the manual work for strategic areas that require human intervention.
Recent innovations in AI and Machine Learning realize the predictive capability which traditional approaches could not. This predictive quality helps manage to anticipate potential problems before being critical, ensuring software quality and reducing risk significantly.
In this article, we would break down the current state of QA Metrics, pointing out the pitfalls, and showcase how AI and ML are transforming the field.
Understanding the Current QA Metrics and Their Limitations
One can not underestimate the role that QA metrics have in terms of measurement of the effectiveness and efficiency of software testing. Commonly applied metrics to this would include defect density, test coverage, and mean time to detect and resolve issues.
As much as these metrics give a snapshot of the testing process, they usually lack in the following:
- First, these are reactive in nature rather than being proactive, as they capture data after the defects are caused, not before.
- Second, these metrics conventionally adopt a naive approach when it comes to contextual issues such as changes in code complexity and user behavior, which in effect affect software quality.
- Last, but not least, such a mechanism for collecting, measuring, and ensuring those metrics is very time-consuming and includes the risk of human error.
This is why beyond such limitations, one has to go ahead with AI and machine learning, which brings out more dynamic and real-time insights in the testing lifecycle.
The Impact of AI and Machine Learning on QA Metrics
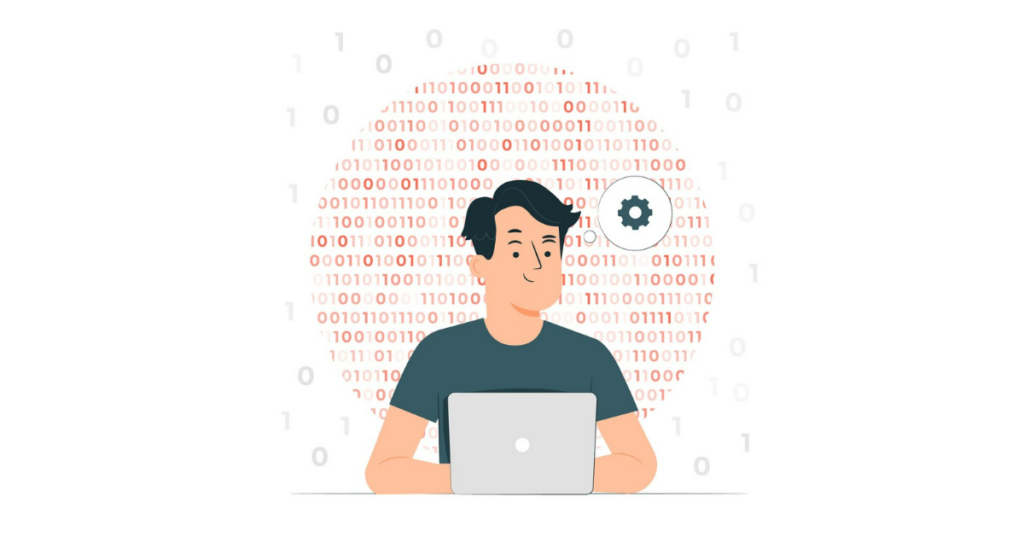
AI and Machine Learning technologies induce another paradigm in QA metrics through predictive and prescriptive analysis.
They allow the analysis of colossal volumes of data in order to understand some form of pattern; which might be at times missed by the human testers.
For example, it will predict that which symptom of code is likely to harbor most of the defects based on history so that testers could pool their custom correctly.
Machine Learning algorithms can increase the test coverage by identifying gaps within the test cases and suggesting the development of new ones.
That way, they can provide more coverage and improve the quality of the software in question. AI-powered tools could continuously monitor the performance of the software being developed, providing real-time metrics to guide speedier decision-making and issue resolution.
AI and machine learning integration in QA metrics, making the testing strategy more informed and proactive, ensuring overall quality and reliability of software products.
Case Studies: Real-world Examples of Improved QA Metrics with AI
A few organizations have managed to bring AI and Machine Learning successfully into QA. For instance, a leading e-commerce company was able to implement an AI-driven testing tool that reduces their defect detection time by 40%.
The tool uses machine learning algorithms to analyze user behavior and make predictions of possible issues before they can affect the customer experience.
The second example is a financial services organization that adopted AI for the purpose of generating and executing automated tests. It became an enabler of increasing test coverage by an additional 30% and saved mitigating manual testing time by a whopping 60%.
The AI engine learned from past defects and continuously improved on its testing strategies to have higher accuracy and reliability.
In this case, the case studies pointed out tangible benefits of using AI and Machine Learning in the improvement of QA metrics with their possible revolution in software testing.
Challenges and Considerations in Adopting AI and Machine Learning in Software Testing
It's all clear with the benefits of AI and Machine Learning in software testing nowadays, but there are a few issues facing it. The first one is related to the quality and availability of the data.
For AI systems to work effectively, they will require massive and high-quality data. Meanwhile, organizations need to make sure the suitable type of infrastructures are in place for these technologies to use.
It can be a challenge to integrate AI and Machine Learning into workflows. It requires a mindset and process change that not all team members may be very receptive to.
Moreover, there is a continuous need for monitoring and updating the AI models so that they can remain accurate and relevant in their respective workflows.
There are other aspects to consider here, the security and ethics concerns; AI systems have to be developed with sensitivity to data supposed to be secured.
And there is a call for transparencies as organizations need to know their space and the well-developed AI level that would be used for their testing processes. Only then can the issues be fixed for the proper uptake of AI and Machine Learning into the process of software testing.
The Future of QA Metrics and Software Testing with AI and Machine Learning
In the future, the QA metrics and software testing done by quality assurance professionals will require the maximum use of AI and ML. These technologies will become much more sophisticated, resulting in much smarter directions and truer predictions.
We might see AI-powered tools that could provide real-time feedback while the code is being written to flag potential issues.
The Machine Learning models primarily focus on the fact that a better ability to adapt to the evolving software environment should be made so that the strategies of testing turn out to be more effective over the period of time.
Besides, using AI in making self-healing test scripts will reduce the maintenance burden that QA teams face, thus freeing up that time for more valuable work.
The future holds the promise of a much more efficient, accurate, and proactive software testing approach powered by the unseen might of AI and Machine Learning.
Recommendations for Implementing AI and Machine Learning in Your QA Process
The following are recommendations for organizations aiming to implement AI and Machine Learning to have their QA process.
Start Small: Get into a pilot project to test the feasibility and benefits of AI-driven testing tools. This helps with getting insights and doing the needful before one goes for scaling up.
Invest in Training: Make sure that your team gets to acquire the skills needed for working with AI and machine learning solutions. You should equip them with training programs and workshops to upscale their capabilities.
Make Data Quality a Top Priority: The quality of data should be high when working with artificial intelligence. Invest in gathering and cleaning data and the processes for quality data management. This should be one of your major focal points in assurance toward feeding the best-possible data into your artificial intelligence models.
Collaborate with Experts: Collaborate with experts in AI and Machine Learning on how to take care of the implementation process. Capitalize on their knowledge and try to wriggle out of falling into the common pitfalls too often.
Monitoring and Updating: Monitor the individual models on a regular basis and make suitable adjustments needed to maintain accurate predictions. There will often be frequent retraining and fine-tuning of models.
Book a Demo and experience ContextQA testing tool in action with a complimentary, no-obligation session tailored to your business needs.
Conclusion
Making these recommendations will allow the organizations to leverage AI and Machine Learning in their quality assurance processes and derive all the associated advantages accruing from speed, accuracy, and dependability.
Undoubtedly, the role now played by AI and Machine Learning in the next dimension of QA metrics and software testing is really shaping up. They afford a means of proactive, economic, and more accurate testing than what was provided for in traditional metrics.
With the use of AI and Machine Learning, an organization can enhance its software quality, reduce time-to-market, and keep up competitiveness in a more dynamic landscape.
Finally, it's time for QA engineers, developers, and project managers to adopt AI-driven testing tools. Kick off a pilot project, invest in training, and ensure data quality to lay a strong groundwork for implementation.
Your QA process deserves more. Sign up for Jasper for free, and unleash the power of AI and Machine Learning today.
Also Read - Building Reliable and Scalable Systems with Site Reliability Engineering (SRE)
We make it easy to get started with the ContextQA tool: Start Free Trial.