Artificial intelligence (AI) is bringing significant transformations across various industries, and the field of quality assurance (QA) is no exception. In this blog we will see AI in Quality Assurance with Augmenting Human Expertise and Not Replacing Testers.
While AI has introduced powerful tools and techniques that enhance testing capabilities, it has also sparked concerns about replacing human testers.
However, it is crucial to recognize that AI is not intended to replace human expertise but rather to augment and complement it.
According to Gartner, over 75% of organizations will incorporate Deep Neural Networks (DNNs) for scenarios where classical Machine Learning (ML) techniques could be applicable.
Pioneering AI adopters have achieved success by employing practical ML solutions to provide tangible business value. Initial projects often relied on conventional statistical machine learning; however, as organizations progressed, they delved into more sophisticated methods, including deep learning, to amplify the influence of AI.
It's crucial to navigate through the hype surrounding AI and understand the full range of options available to effectively address business challenges. Prioritize simplicity over popular yet complex choices.
Role of AI in Quality Assurance
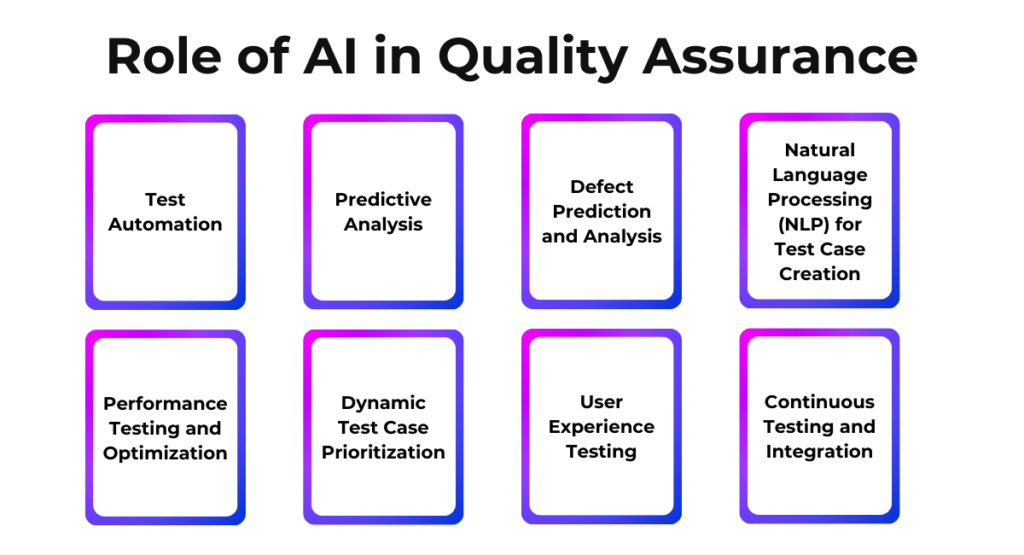
AI can significantly enhance QA processes by automating repetitive tasks, identifying patterns and anomalies, and predicting potential issues.
This automation frees up human testers to focus on more strategic and creative aspects of testing, such as exploring complex test scenarios, evaluating user experience, and making informed decisions based on data-driven insights.
Test Automation:
AI contributes significantly to test automation, enabling the creation and execution of test scripts with greater efficiency. Machine learning algorithms can learn from test outcomes, identifying patterns and optimizing test scenarios over time.
This enhances the speed and accuracy of testing processes, especially in large and complex software projects.
Predictive Analysis:
AI facilitates predictive analysis in QA by examining historical data, identifying trends, and predicting potential issues before they arise. This proactive approach helps in preventing defects and optimizing the overall quality of the software.
Defect Prediction and Analysis:
AI algorithms can analyze code changes, historical defect data, and other parameters to predict potential areas where defects might occur. This assists QA teams in focusing their efforts on critical areas, reducing the likelihood of defects in the final product.
Natural Language Processing (NLP) for Test Case Creation:
NLP capabilities in AI are employed to convert natural language requirements into executable test cases. This streamlines the test case creation process and ensures that test cases are aligned with the intended functionality of the software.
Performance Testing and Optimization:
AI helps in performance testing by simulating user behavior and identifying potential bottlenecks in the system. Machine learning algorithms can optimize performance testing scenarios based on real-time data, improving the efficiency of performance testing processes.
Dynamic Test Case Prioritization:
AI can dynamically prioritize test cases based on the code changes, historical defect data, and the criticality of specific features. This ensures that high-priority test cases are executed first, providing faster feedback on critical functionalities.
User Experience Testing:
AI plays a role in user experience testing by automating the evaluation of user interfaces, identifying potential usability issues, and ensuring a seamless and intuitive user experience.
Continuous Testing and Integration:
AI supports continuous testing and integration practices by automating the testing of code changes in real-time. This enables rapid feedback loops, ensuring that issues are identified and addressed early in the development process.
Specific Actionable Applications of AI in Quality Assurance
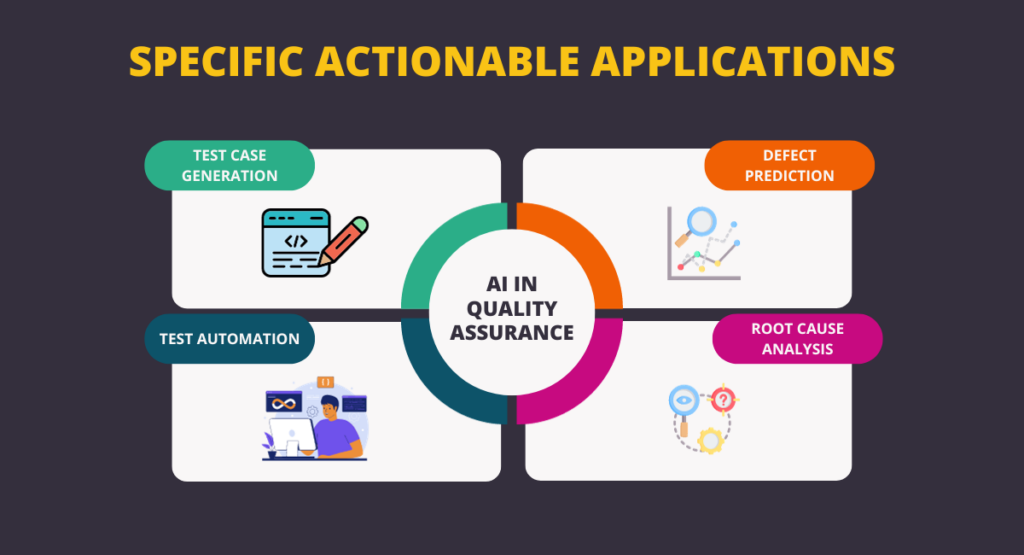
Test Case Generation:
AI algorithms can analyze historical test data and user behavior to generate new test cases that cover a wider range of scenarios and potential issues.
Defect Prediction:
AI models can identify patterns in code and historical defects to predict areas of the codebase that are more likely to contain bugs. This enables testers to prioritize their efforts and focus on areas with a higher risk of defects.
Test Automation:
AI-powered tools can automate various testing tasks, such as regression testing, API testing, and performance testing. This automation reduces the time and effort required to execute repetitive tests, allowing testers to focus on more complex and exploratory testing activities.
Root Cause Analysis:
AI can analyze test results and identify underlying causes of defects, providing testers with valuable insights to prevent similar issues from recurring.
In February 2023, Kruger Packaging, a Canadian company producing furniture items from recycled materials, allocated approximately US$22 million to embrace technological advancements.
This investment encompasses the adoption of new technologies such as AI-assisted quality control and emission control technology.
Book a Demo and experience ContextQA testing tool in action with a complimentary, no-obligation session tailored to your business needs.
Superhuman
AI is not a threat to the QA profession; it is an opportunity to elevate it to new heights. By leveraging AI's capabilities to automate routine tasks and uncover hidden insights, human testers can focus on their core strengths of creativity, critical thinking, and problem-solving.
This collaborative approach between humans and AI will revolutionize the QA landscape, leading to the development of more reliable, secure, and user-friendly software products.
You may also be interested in: QA vs. SDET: Contrasting Approaches to Software Quality Assurance